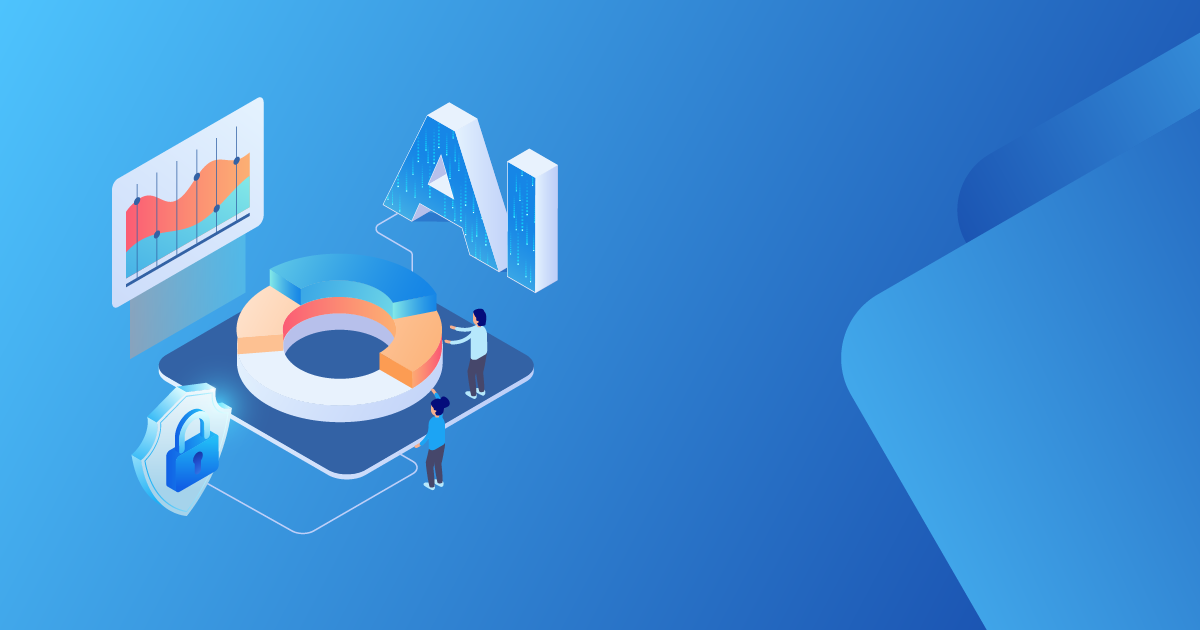
AI-Based Payment Analytics: Enhancing Fraud Detection and Automating Transactions
March 30, 2025
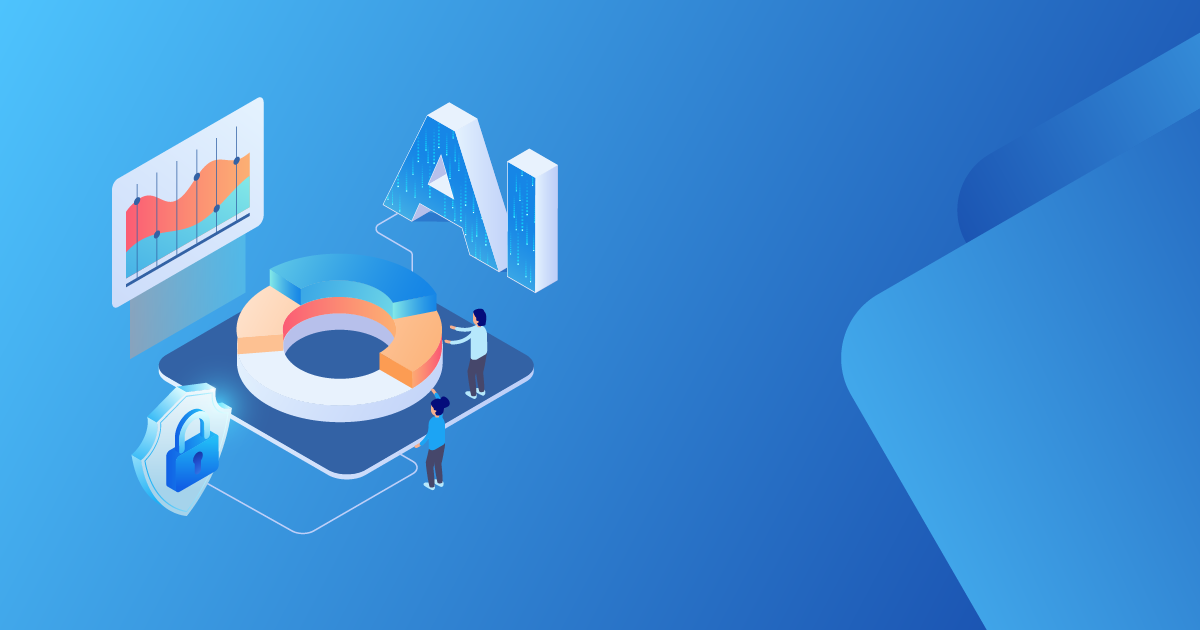
In today’s evolving financial landscape, managing the growing volume of transactions requires smarter solutions. AI-based payment analytics provide the promising answer of real-time insights, offering two main functions: fraud detection through sophisticated reasoning and automating routine payments based on historical patterns and forecasts.
Two-Fold Approach to Payment Analytics
At the heart of AI payment solutions is a dual capability: identifying anomalies and automating standard payments. Anomaly detection focuses on identifying unusual patterns, not just fraud. Many fraud alerts are false positives—transactions that deviate from historical patterns but aren't necessarily fraudulent. These AI systems flag suspicious activities and explain why they’re considered outliers. This reasoning is key, providing business users with clear insights into the factors behind each alert, such as deviations in transaction amounts, unusual payment timings or uncommon combinations of creditor and debtor relationships.
On the other hand, payment automation leverages historical data and predictive analytics. The system learns which transactions are routine, such as recurring payments like subscriptions or supplier invoices, which follow predictable patterns. With accurate forecasting, it can automate these transactions without human intervention, reducing administrative overhead and minimizing errors. AI recognizes patterns from past data and adapts to any changes, making informed decisions to process transactions automatically.
Role of Reasoning in AI-Payment Analytics
One of the most critical aspects of AI-based payment analytics is providing clear explanations for its decisions. Business users need more than a simple alert; they need to understand why a transaction deviates from the norm. This is where reasoning comes in. Instead of a black-box approach, modern systems offer transparent, rule-based insights. For example, if a payment is flagged as anomalous, the system might explain that it deviates from the typical amount, occurs at an unusual time, or involves a creditor-debtor relationship that doesn’t align with similar transactions. This transparency builds trust and allows users to refine their processes, ensuring genuine transactions aren’t mistakenly blocked while suspicious ones are reviewed.
Adaptability: Building New Patterns in Real Time
Payment patterns are not static, they evolved due to market dynamics, changes in consumer behavior, regulatory shifts and seasonal factors. AI analytics solutions must be adaptable, capable of learning and updating their models in real time. This ensures the system remains relevant as payment behaviors shift.
Think of the system as a team of experts, each focusing on different aspects of payment history. One may analyze creditor-debtor relationships, another the frequency and volume of transactions, and another the timing and amounts. By combining these insights, the system builds a dynamic understanding of what “normal” looks like. When a new transaction occurs, it is evaluated against this historical context and any deviation is flagged with an explanation reflecting input from these experts.
Real-World Applications
- Fraud Detection in E-Commerce:
Online retailers process thousands of transactions daily. An AI system monitors these in real time, flagging anomalies such as sudden spikes in purchase amounts or atypical payment patterns. For example, if a customer who typically makes small purchases initiates a high-value order, the system alerts the fraud department and provides reasoning, such as deviation from the customer’s usual spending behavior or unusual payment timing. - Automated Invoice Payments for Businesses:
Businesses often have recurring payments for services and supplies. An AI solution learns the historical patterns of these transactions and automates processing when the details match. If an invoice closely resembles past transactions in amount, timing and creditor-debtor relationship, the system approves and processes it automatically. Deviations trigger a manual review with an explanation for the difference. - Banking Operations and Check Clearing:
Banks use real-time analytics not just for fraud prevention but to improve operational efficiency. In check clearing, the system analyzes historical data to establish normal processing times, amounts and parties involved. If a check deviates from these parameters, it is flagged for review, ensuring routine transactions proceed automatically while anomalies are scrutinized, reducing delays and operational risk.
The Bottom Line
Real-time transaction analysis using AI is transforming the payments landscape. Whether through precise anomaly detection or seamless automation of routine transactions, these solutions are a game-changer for organizations handling large volumes of payments. The combination of real-time adaptability, multi-expert reasoning and transparency in decision-making helps businesses mitigate risks while optimizing efficiency.
The shift to intelligent systems goes beyond reducing false positives or automating tasks—it empowers businesses with actionable insights. As payment patterns evolve, so must the tools that analyze them. With AI-based payment analytics, organizations are better equipped to navigate an ever-changing financial environment, ensuring security and efficiency are maintained at the highest levels.